Unleashing Potential: The Importance of Image Datasets for Object Detection
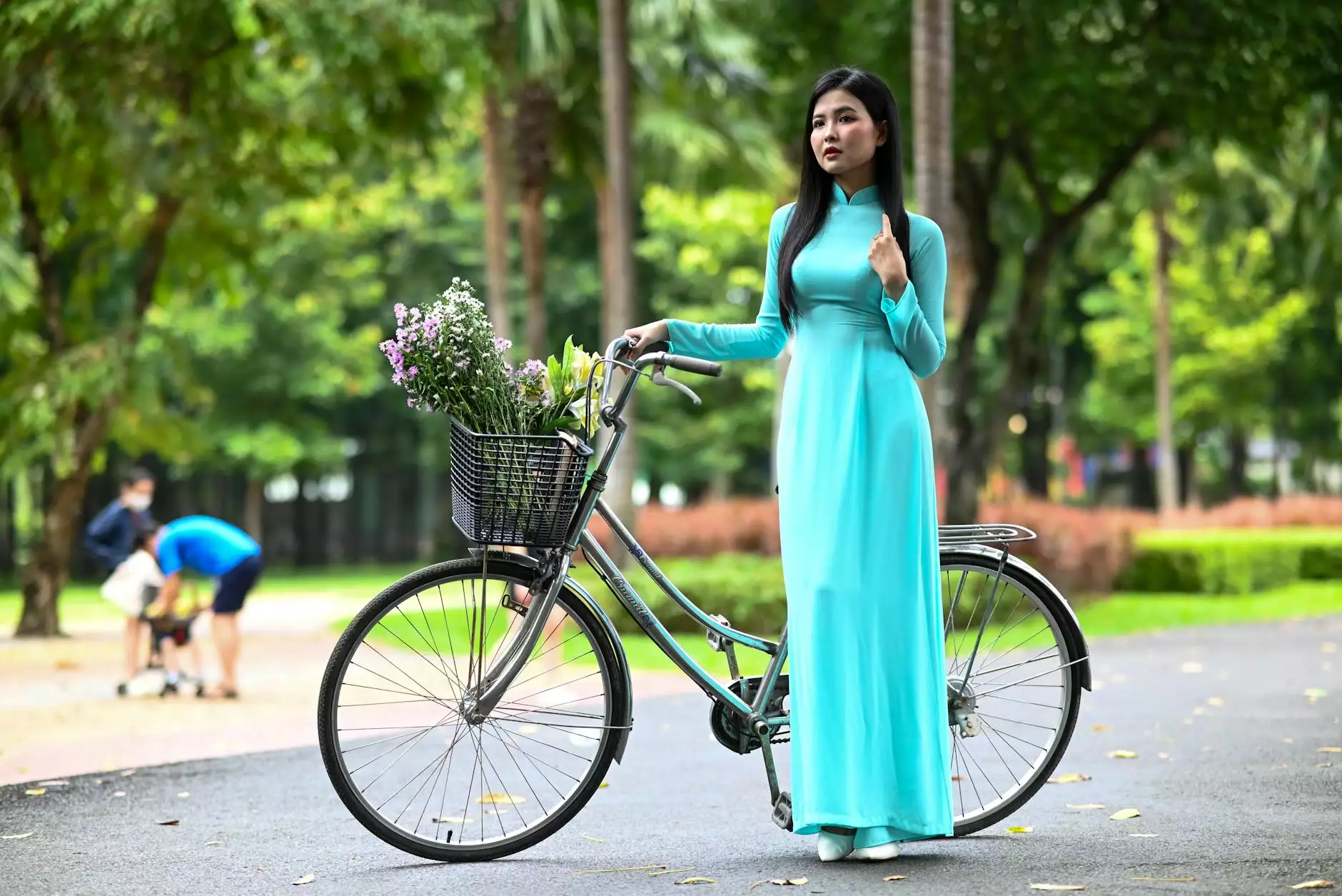
The realm of artificial intelligence (AI) is rapidly evolving, with innovations like object detection becoming pivotal in various fields, from autonomous vehicles to security surveillance. Central to the success of these advanced technologies is the necessity for high-quality, rich image dataset for object detection. This article delves deep into the significance of image datasets, the process of data annotation, and how platforms such as Keylabs.ai are redefining the way we gather and analyze visual data.
Understanding Image Datasets in Object Detection
Image datasets serve as the foundation for training algorithms, particularly in object detection, a subfield of computer vision. These datasets are collections of images that include annotated information for various objects within each image. This annotation process allows machine learning models to understand and recognize objects, leading to improved accuracy in detection tasks.
What is Object Detection?
Object detection is the technological process of identifying instances of objects of a certain class in images or videos. This process typically involves two core aspects:
- Object Localization: Determining where an object is located in an image.
- Object Classification: Identifying the class of the detected object.
For a machine learning model to achieve remarkable performance, it requires a diverse and extensive image dataset for object detection. These datasets help the algorithms learn various object features and variations across different conditions such as lighting, angles, and background distractions.
The Role of Data Annotation in Building Image Datasets
Data annotation is the crucial process of labeling data, in this case, images, to prepare them for training AI models. Without proper annotation, an image dataset cannot serve its purpose effectively. The quality and consistency of annotations greatly influence the performance of object detection models.
Types of Data Annotation in Object Detection
There are several methods of data annotation, each serving unique purposes:
- Bounding Box Annotation: Drawing a bounding box around the object of interest to indicate its presence and location.
- Polygon Annotation: For complex shapes that cannot be enclosed in a simple rectangle, polygon annotations offer a more accurate depiction.
- Segmentation Annotation: This involves classifying each pixel in an image to provide a detailed understanding of the object’s shape and form.
- Keypoint Annotation: Identifying critical points in an object, which is commonly used for human pose estimation.
How High-Quality Image Datasets Elevate Object Detection Outcomes
The success of object detection technologies relies significantly on the quality of image datasets used for training. Here are essential factors that describe how high-quality datasets improve outcomes:
Diversity and Variability
A well-rounded image dataset for object detection must include a wide variety of samples, encompassing:
- Different Lighting Conditions: Images taken in bright, dim, or mixed lighting to ensure robustness.
- Various Angles and Perspectives: Objects should be captured from multiple viewpoints.
- Different Backgrounds and Contexts: Inclusion of diverse environments helps in reducing background noise.
This diversity enables AI models to generalize knowledge, leading to better accuracy in real-world applications.
Accuracy of Annotations
The precision of annotations directly impacts model accuracy. Mislabeling or poor-quality annotations can lead to significant errors during training, which can propagate into performance issues. Hence, employing specialized data annotation tools is crucial for maintaining high standards.
Leveraging Data Annotation Tools and Platforms
To create rich image datasets for object detection, organizations must invest in data annotation tools that ensure efficiency and precision. Keylabs.ai offers state-of-the-art data annotation platforms designed to cater to your specific needs in the realm of object detection.
Features of Keylabs.ai Data Annotation Platform
Keylabs.ai stands out with a multitude of features tailored to streamline the data annotation process:
- User-Friendly Interface: Simplifies the annotation workflow, making it accessible to users of all technical backgrounds.
- Advanced Annotation Tools: Various tools such as bounding boxes, polygon, and segmentation options facilitate different types of annotations needed for comprehensive datasets.
- Collaboration Features: Allows multiple users to work on annotation projects simultaneously, enhancing productivity.
- Quality Control Mechanisms: Built-in checks ensure that annotations meet predefined quality standards.
- Scalability: Accommodates projects of any size, from small datasets to extensive image collections.
Case Studies: Success Stories of Object Detection Using Quality Datasets
Real-world applications of object detection showcase the profound effects of utilizing high-quality image datasets. Here are a few notable instances:
Autonomous Vehicles
Companies like Tesla and Waymo rely heavily on image datasets for object detection to enhance the functionalities of their vehicles. By training their systems on diverse datasets, they significantly minimize the chances of misidentifying pedestrians, cyclists, and other vehicles, which enhances safety in autonomous driving scenarios.
Healthcare Applications
Health tech firms are utilizing object detection to identify tumors in medical imaging. High-resolution image datasets annotated to highlight areas of interest lead to better diagnostic capabilities, proving essential for early detection and treatment of diseases.
Facial Recognition Systems
Facial recognition technologies have advanced considerably, primarily due to the availability of extensive image datasets. These datasets have allowed models to learn variations in human faces, leading to improved accuracy in identification and security systems.
Challenges in Image Dataset Creation for Object Detection
Despite the necessity of high-quality image datasets, several challenges hamper their creation:
Cost and Time Constraints
Compiling a substantial image dataset requires considerable investment in time and resources, including acquiring images and ensuring high-quality annotations.
Data Privacy Concerns
Gathering images featuring individuals raises data privacy issues, necessitating strict adherence to regulations like GDPR.
Consistency in Annotation
Maintaining annotation consistency across large collaborations can be difficult, leading to variations in quality. This underscores the importance of using effective data annotation platforms that incorporate quality control features, like those available at Keylabs.ai.
The Future of Image Datasets for Object Detection
As technologies continue to evolve, so too will the methods for creating and utilizing image datasets for object detection. Innovations such as synthetic data generation and augmented reality are paving the way for sustainable data collection practices that can alleviate some of the challenges outlined previously.
Synthetic Data Generation
Synthetic data generation involves creating artificial data using algorithms and simulations. These datasets can be customized to include specific object types and diverse conditions without the limitations of real-world data collection.
Augmented Reality for Dataset Expansion
Augmented reality (AR) technologies can assist in creating datasets by overlaying digitally rendered objects into real-world environments, allowing for rich interactions and real-time data collection.
Conclusion
In conclusion, the importance of a quality image dataset for object detection cannot be overstated. The advancements in AI rely heavily on the integrity and richness of these datasets. By leveraging platforms like Keylabs.ai that offer cutting-edge data annotation tools, businesses can significantly enhance their AI models' performance and accuracy.
As the landscape of object detection continues to advance, investing in high-quality image datasets coupled with effective annotation processes will remain integral to harnessing the full potential of artificial intelligence.
Get Started with Keylabs.ai
Are you ready to take your AI capabilities to the next level? Explore the exceptional data annotation tools and platforms at Keylabs.ai today. Your journey towards creating superior image datasets for object detection begins here.