Understanding the Learning System Model in Machine Learning: Unlocking Business Potential through Intelligent Data Strategies
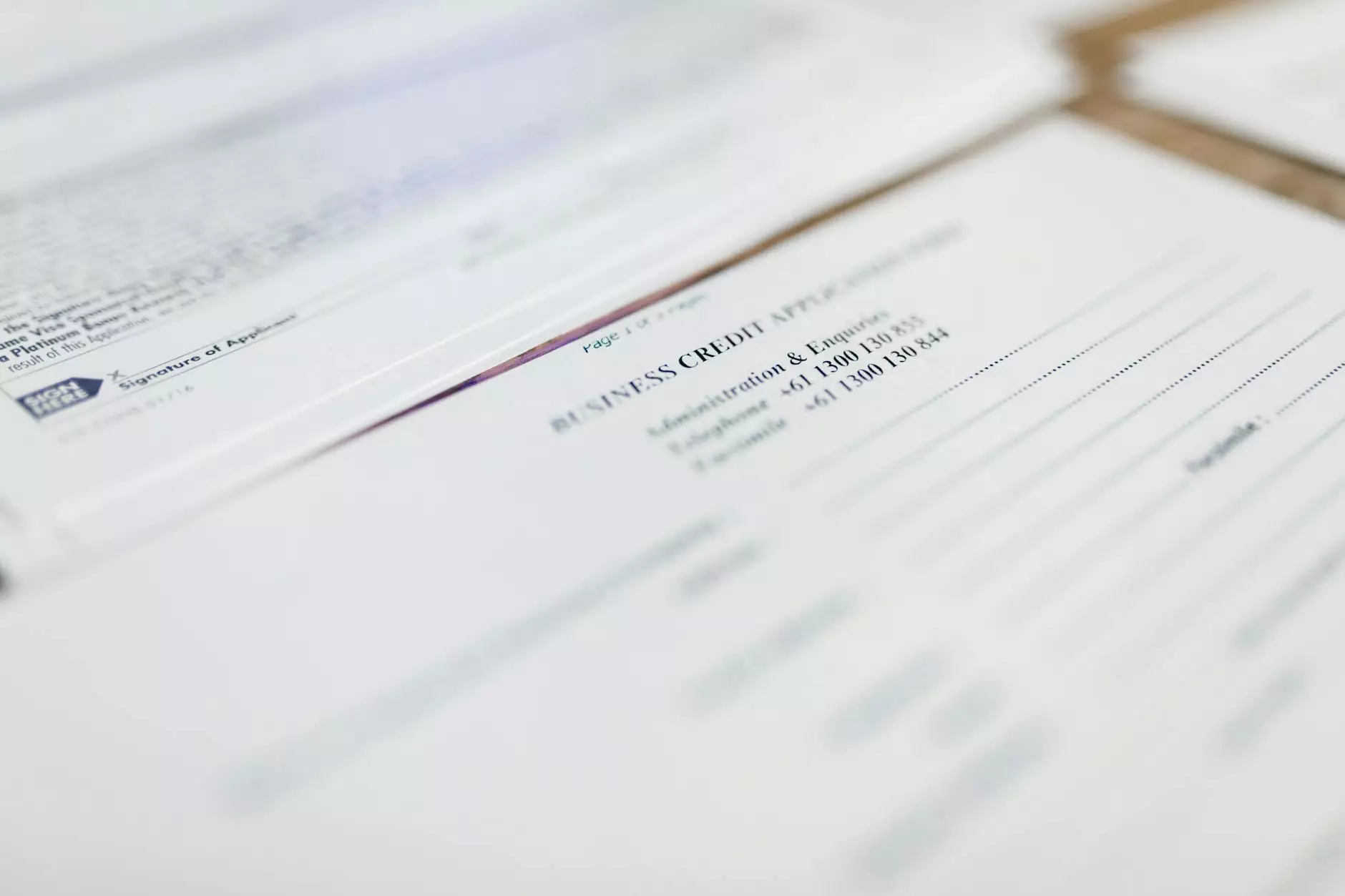
In today's rapidly evolving digital economy, businesses are constantly seeking innovative ways to leverage data to enhance operational efficiency, improve decision-making, and deliver superior customer experiences. At the heart of these advancements lies the learning system model in machine learning, a foundational concept that enables organizations to build intelligent systems capable of learning from data, adapting to new information, and autonomously improving performance over time.
Introduction to the Learning System Model in Machine Learning
The learning system model in machine learning refers to a structured framework that defines how machines learn from data, make predictions, and refine their processes to achieve desired outcomes. This model encapsulates the entire lifecycle of machine learning, from data collection and preprocessing to model training, evaluation, and deployment.
Understanding this model is crucial for businesses aiming to implement advanced data strategies such as content management, automation of business processes, and robust data governance systems. When effectively harnessed, the learning system model can transform raw data into valuable insights, enabling companies like Intalio to stay ahead in competitive markets.
The Core Components of the Learning System Model
The learning system model in machine learning comprises several essential components that work together to facilitate effective learning and decision-making:
- Data Acquisition: Gathering relevant, high-quality data from various sources such as transaction logs, social media, sensors, and databases.
- Data Processing and Preprocessing: Cleaning, transforming, and organizing data to ensure it is suitable for model training.
- Feature Extraction and Selection: Identifying the most relevant variables that influence outcomes and reduce noise in the data.
- Model Selection and Training: Choosing the appropriate machine learning algorithms and training them on prepared datasets to learn patterns.
- Model Evaluation and Validation: Testing the trained models against unseen data to assess accuracy, robustness, and generalization capabilities.
- Deployment and Integration: Implementing trained models into real business environments where they can make autonomous predictions and decisions.
- Monitoring and Maintenance: Continuously tracking model performance over time and updating models as data evolves or new patterns emerge.
Each of these components plays a vital role in creating a resilient and adaptable machine learning system that can significantly enhance business processes.
Applying the Learning System Model in Business: A Strategic Perspective
Implementing the learning system model in machine learning within a business context provides tangible benefits that span multiple operational domains:
Optimizing Content Management Services
In the realm of content management services, machine learning models powered by the learning system framework enable businesses to automate content classification, tagging, and personalization. For example, intelligent content recommendation engines analyze user interaction data to deliver highly relevant content, thereby enhancing engagement and conversion rates.
Enabling Business Process Automation Services
Automation is at the core of modern enterprise efficiency. The learning system model in machine learning facilitates intelligent automation by enabling systems to learn from operational data and optimize workflows without human intervention. This leads to faster decision cycles, reduced operational costs, and increased accuracy in routine tasks such as invoice processing, customer onboarding, and supply chain management.
Enhancing Data Governance Systems
Robust data governance systems depend on machine learning to ensure data quality, compliance, and security. Learning models can automatically detect anomalies, classify sensitive information, and monitor data access patterns, thereby reinforcing regulatory adherence and securing enterprise data assets.
Technological Foundations Supporting the Learning System Model
Several key technologies underpin the effective implementation of the learning system model in machine learning:
- Big Data Infrastructure: Scalable systems to store and process massive datasets efficiently, such as Hadoop, Spark, and cloud-based solutions.
- Machine Learning Frameworks: Libraries and platforms like TensorFlow, PyTorch, and scikit-learn enable rapid development and deployment of models.
- Artificial Intelligence and Deep Learning: Advanced techniques that improve pattern recognition and predictive accuracy for complex data types like images, text, and speech.
- Automation Tools: Robotic Process Automation (RPA) and orchestration tools automate routine tasks based on machine learning insights.
These technological enablers make it possible for organizations to develop sophisticated learning systems that align with their strategic goals, thereby transforming raw data into a competitive advantage.
Challenges and Best Practices in Implementing the Learning System Model
While the benefits are numerous, deploying a learning system in machine learning presents several challenges:
- Data Quality and Quantity: Insufficient or poor-quality data hampers model accuracy.
- Model Interpretability: Complex models may behave as black boxes, making it difficult to understand their decision-making processes.
- Ethical and Legal Considerations: Ensuring compliance with data privacy laws and avoiding bias in models.
- Scalability: Maintaining system performance as data volume and complexity grow.
To navigate these challenges effectively, organizations should adhere to best practices such as establishing clear data governance policies, investing in talent and training, conducting thorough model validation, and fostering an organizational culture that values ethical AI use.
The Future of Business with Learning System Models in Machine Learning
The future of enterprise competitiveness hinges on the ability to adopt and adapt advanced learning system models in machine learning. As technologies evolve, we can anticipate developments such as:
- AutoML (Automated Machine Learning): Automating the end-to-end process of model development to democratize AI access.
- Edge AI: Deploying learning models directly on devices for real-time analytics and decision-making, enhancing latency and privacy.
- Explainable AI: Developing transparent models that provide insights into their decision processes, improving trust and compliance.
- Integrated Data Ecosystems: Seamless data integration across organizational silos to enable holistic learning systems.
Companies like Intalio are pioneering these trends by offering tailored solutions that embed machine learning into content management, automation, and data governance, driving innovation and sustainable growth.
Leveraging the Learning System Model for Business Transformation
To fully harness the potential of the learning system model in machine learning, organizations should consider the following strategic actions:
- Invest in Data Infrastructure: Build scalable, secure, and interoperable data environments.
- Develop Talent and Capabilities: Upskill teams in AI, data science, and analytics.
- Foster a Culture of Innovation: Encourage experimentation with machine learning solutions across departments.
- Partner with Experts: Collaborate with technology providers who specialize in AI-driven enterprise solutions.
By doing so, businesses can create adaptive, intelligent systems that not only optimize existing processes but also open new avenues for growth and value creation.
Conclusion: Embracing the Future of Business with Machine Learning Systems
The learning system model in machine learning embodies a transformative approach that equips businesses with the tools to transform raw data into strategic assets. When integrated into content management, process automation, and data governance, this model becomes a catalyst for innovation, operational excellence, and competitive differentiation.
As technology continues to advance, organizations committed to leveraging these models will position themselves at the forefront of economic progress, establishing resilient, intelligent frameworks capable of navigating the complexities of the digital age. Partnering with expert providers like Intalio ensures that your business is equipped with cutting-edge solutions tailored to your unique needs, ultimately driving sustainable success.